CUHK ARTS strives to be one of the best higher education institutions in Asia committed to research in the arts and humanities with global impact. Our academic staff is well-positioned to undertake cutting-edge interdisciplinary scholarship and collaborations.
Global
Innovative
Inspirational
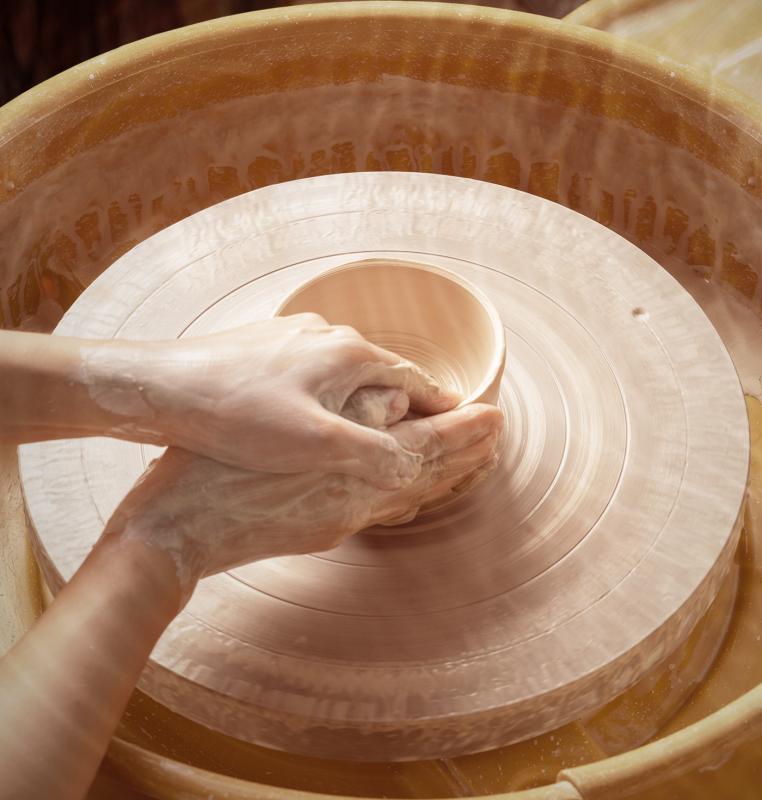
[Call for Entries!] CUHK Poetry Contest 2025!
All CUHK students enrolled in the 2025 – 26 academic year are welcome to join this exciting event. Submit your poem online by 3 October, Friday (12noon, Hong Kong Time). For enquiries, please contact airp@cuhk.edu.hk.
Guidance

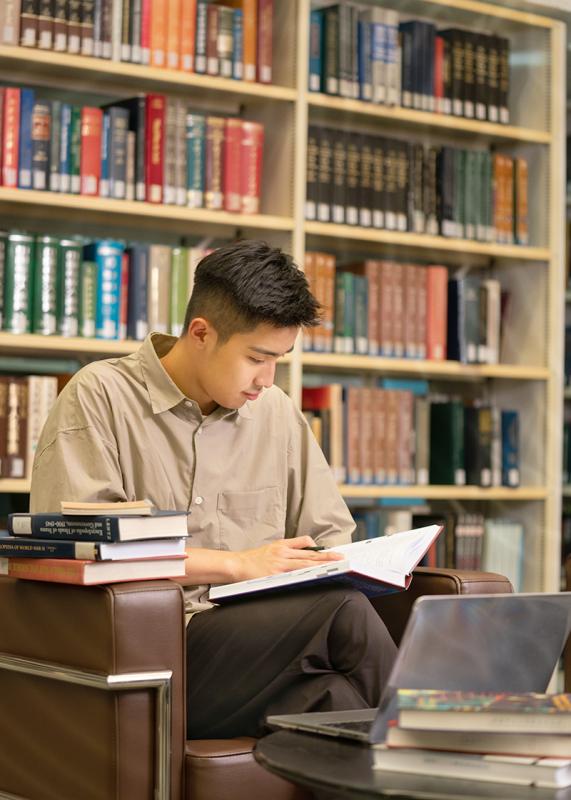
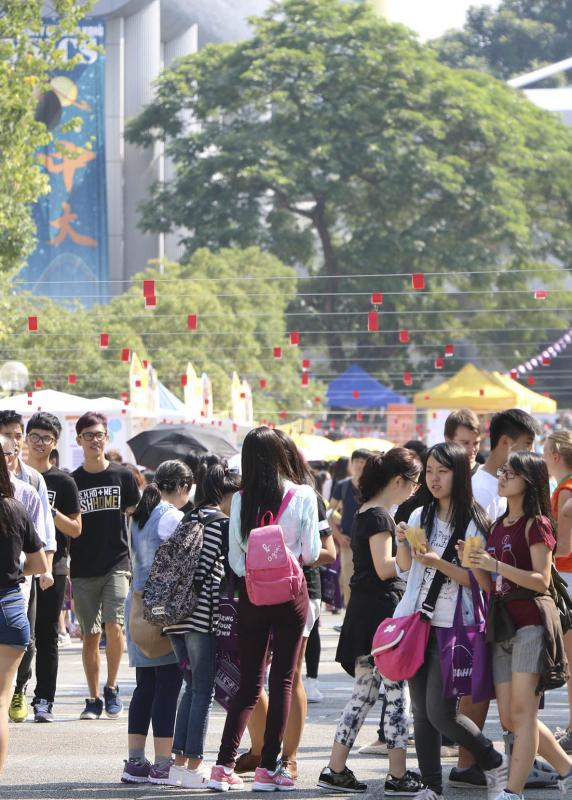
I am a
Student
Scholar
Member of the Public
- Why do I need to study at CUHK ARTS?
- Which programmes are suitable for me?
- What scholarships and awards can I apply for?
- Will I get academic advice?
Research & Scholarship
We are committed to bridging Chinese and Western traditions with innovative research.
As humanities scholars, we seek not only to contribute new knowledge and ideas, but also to become insightful and engaging educators.
Explore Research and Scholarship
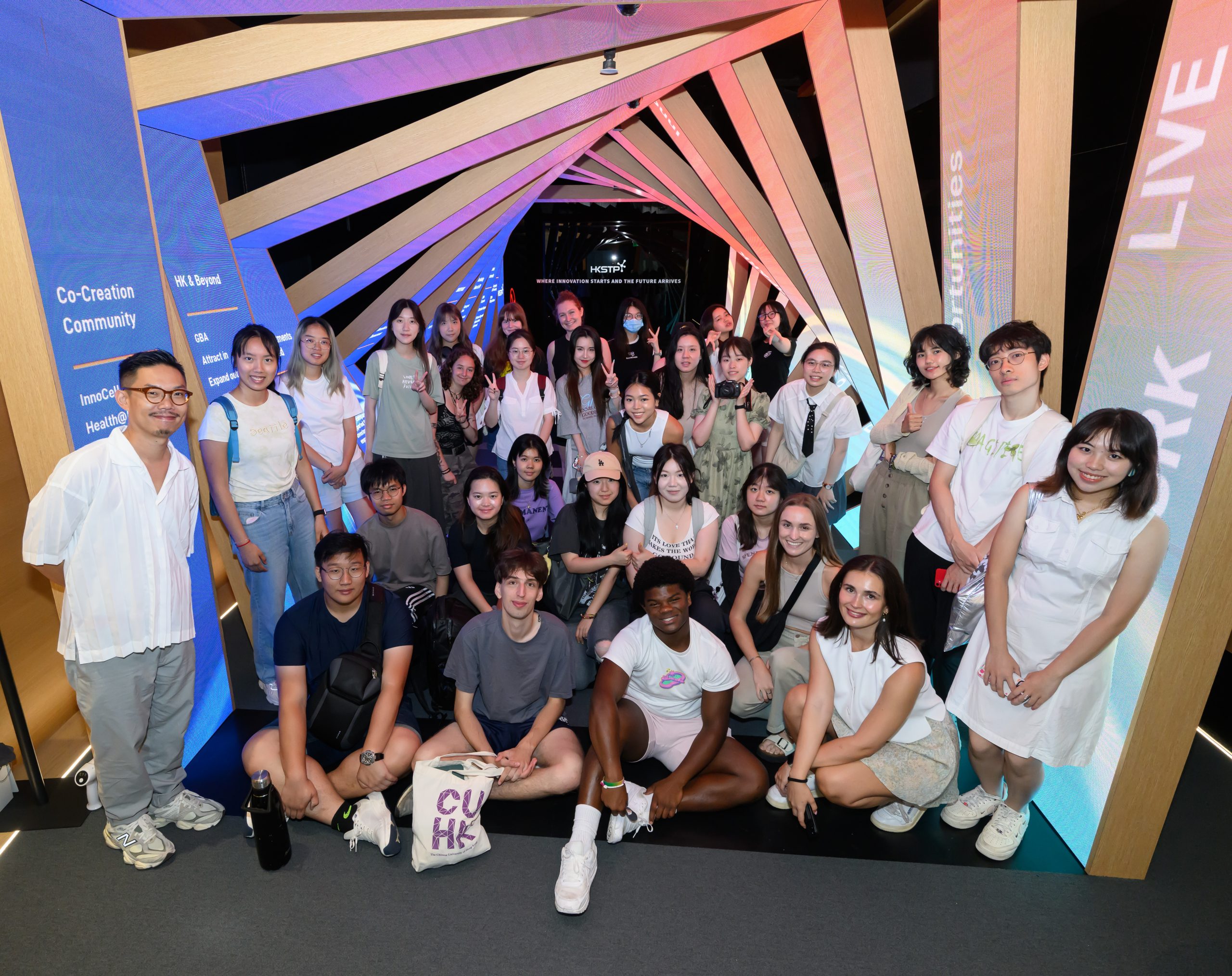
One Step Closer
Explore More Stories
Stories
The way to bring deaf and hearing people closer together – Film screening celebrates inclusiveness at CUHK
Stories
The way to bring deaf and hearing people closer together – Film screening celebrates inclusiveness at CUHK
The cast and crew of The Way We Talk, including director Adam Wong and actress Chung Suet-ying, and CUHK Professor Gladys Tang who supported the team with her work on deaf education, share their thoughts at the post-screening discussion
Professor Gladys Tang
Linguistics and Modern Languages
Explore More
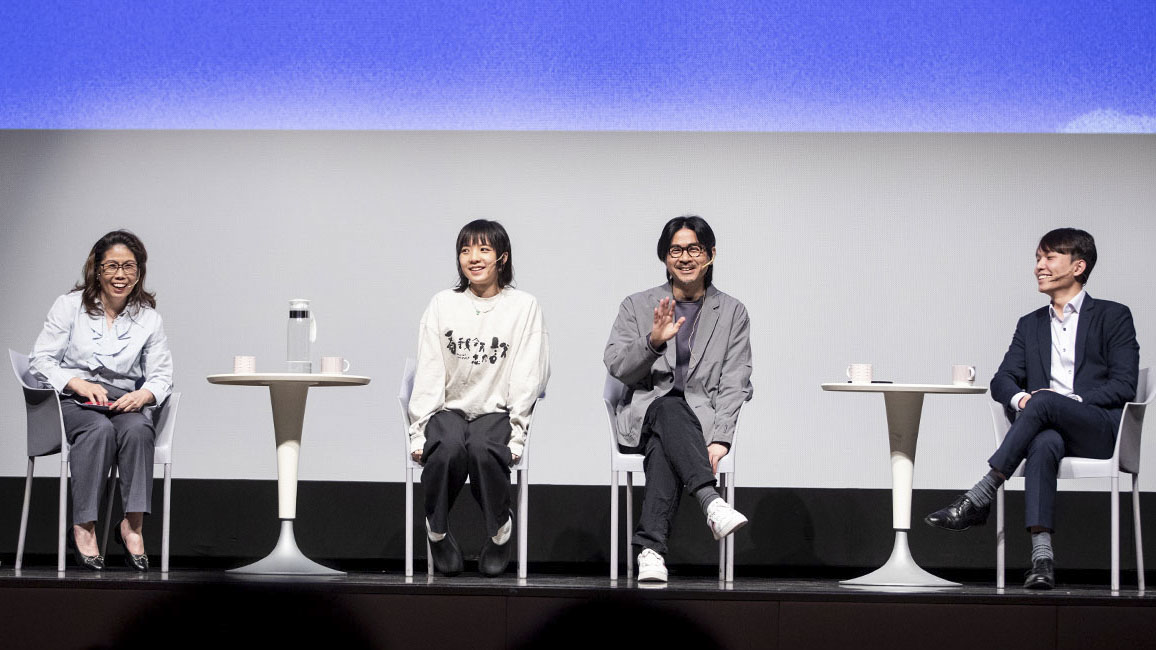
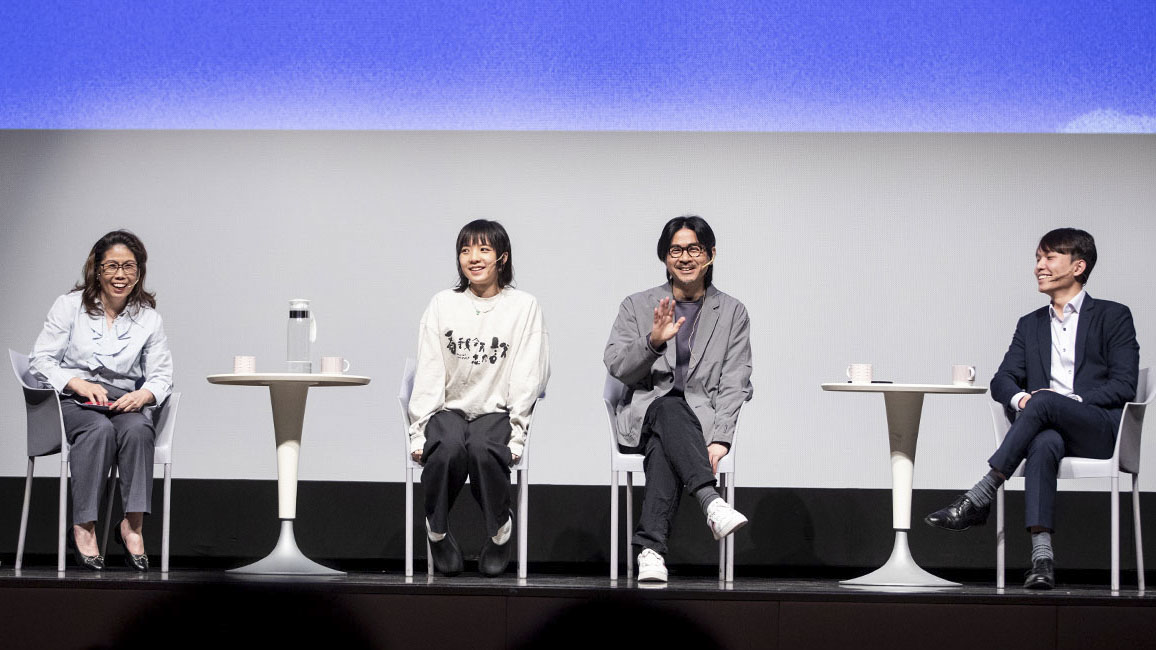
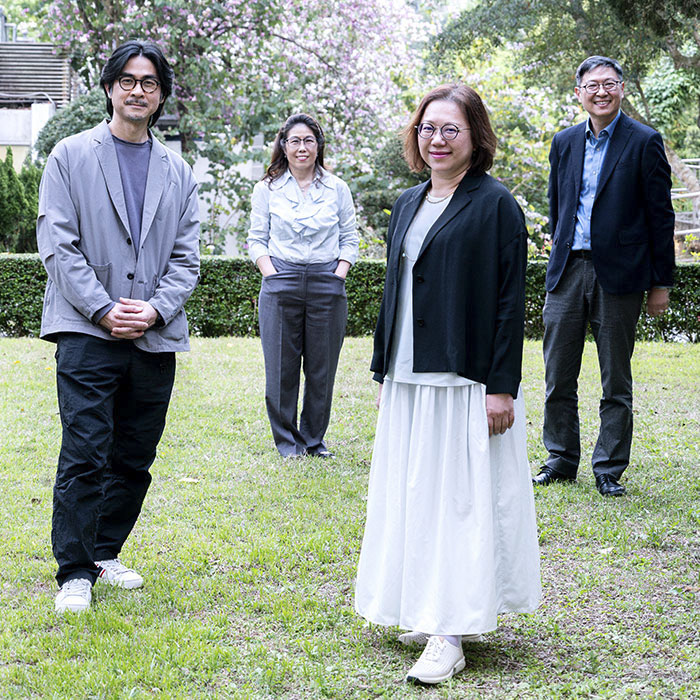
Stories
Art of documentary photography – Prize-winning photojournalist Liu Heung Shing has a date with CUHK in October
Stories
Art of documentary photography – Prize-winning photojournalist Liu Heung Shing has a date with CUHK in October
Over the last half a century, Pulitzer Prize-winning photojournalist Liu Heung Shing has been globetrotting with a camera and press pass to chronicle through his lens the first rough drafts of history.
Liu Heung Shing
/
Explore More
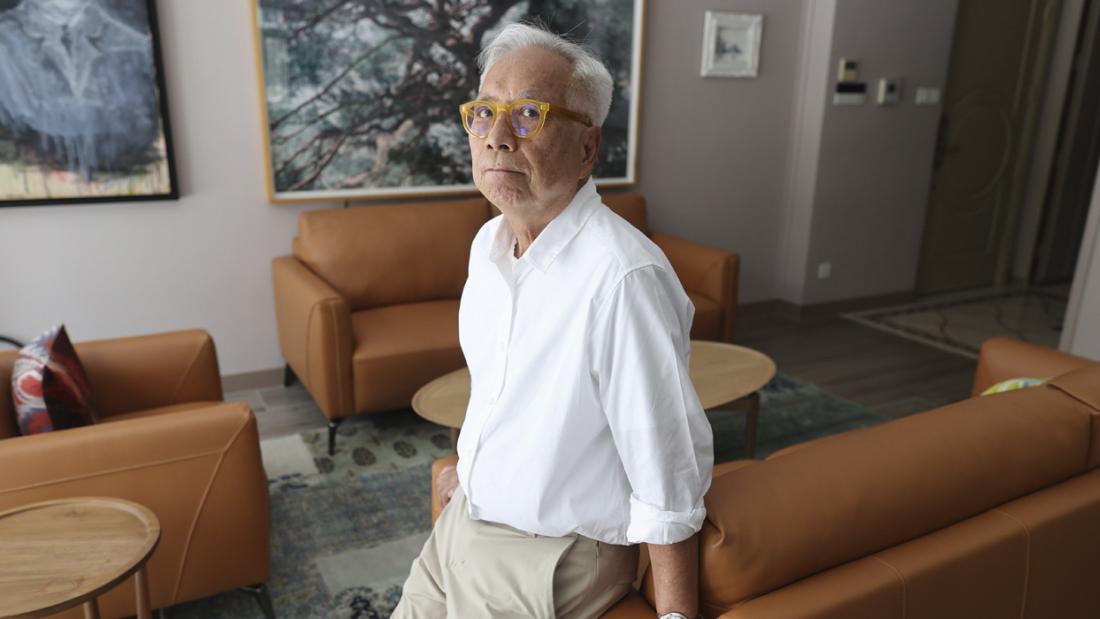
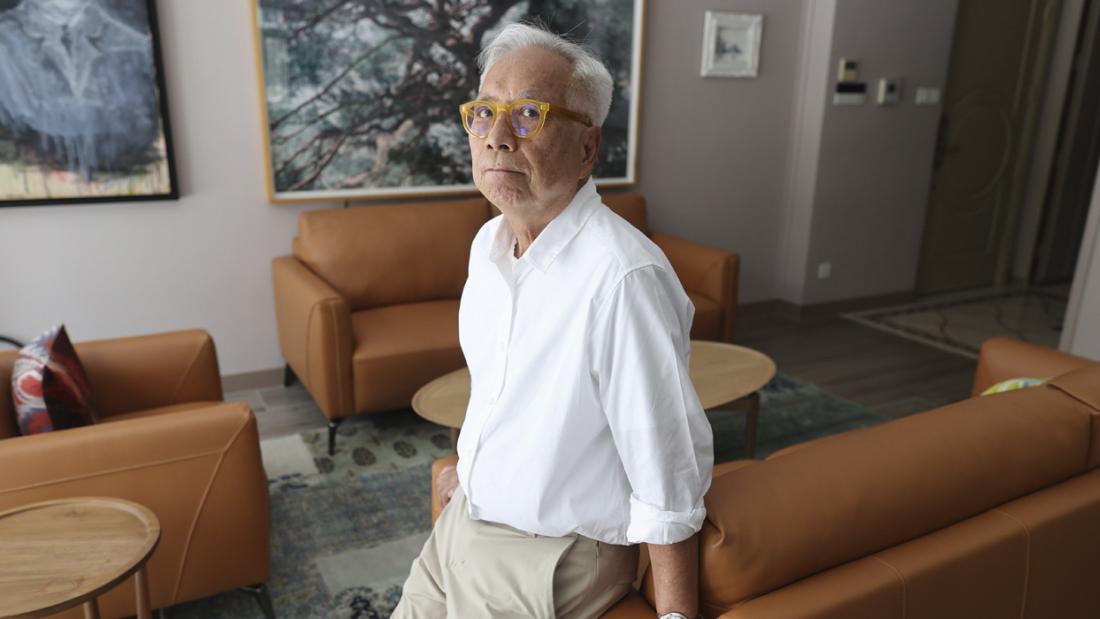
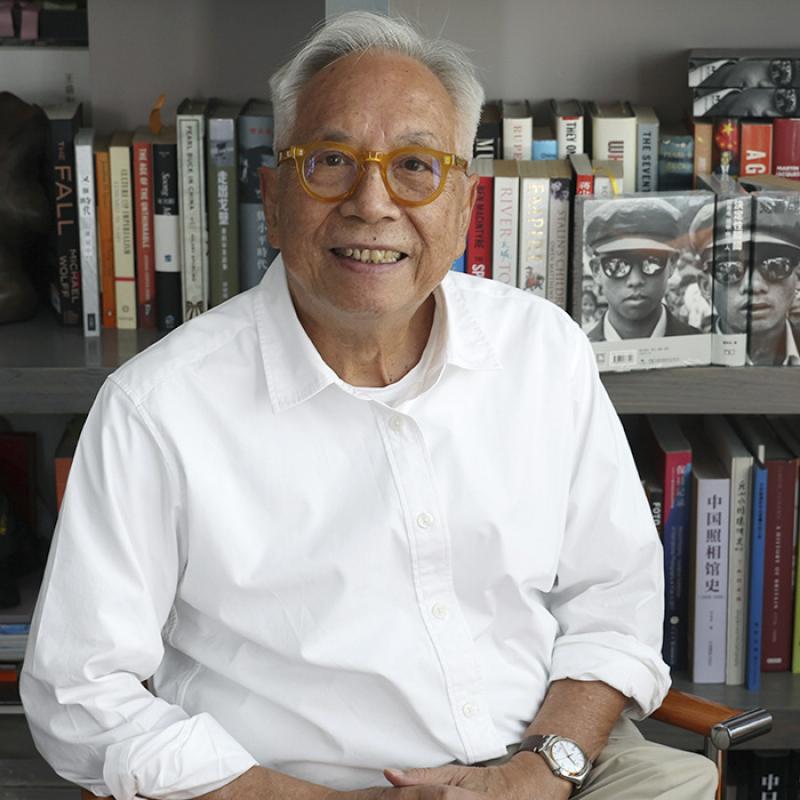
Stories
A True Renaissance Man
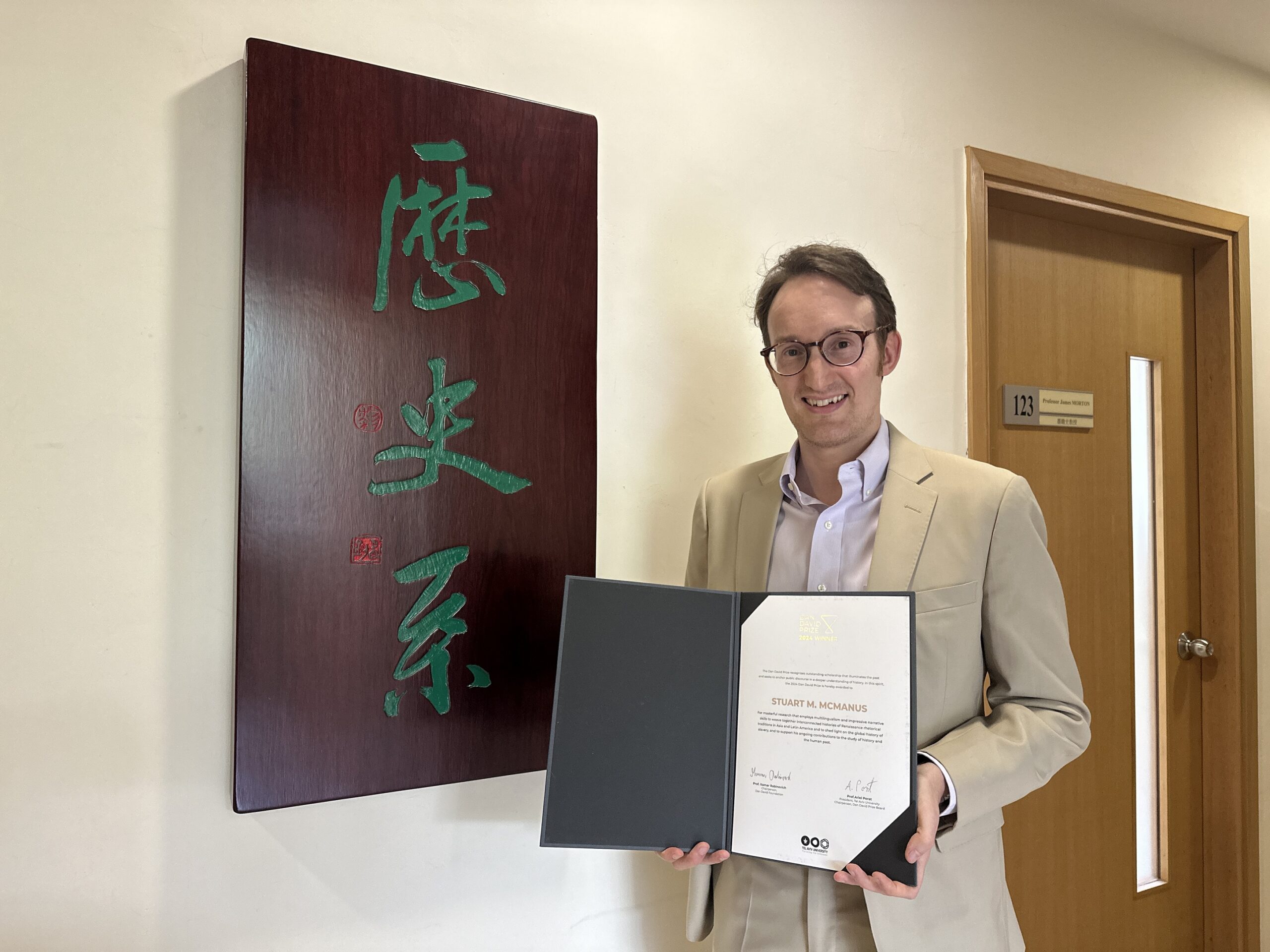
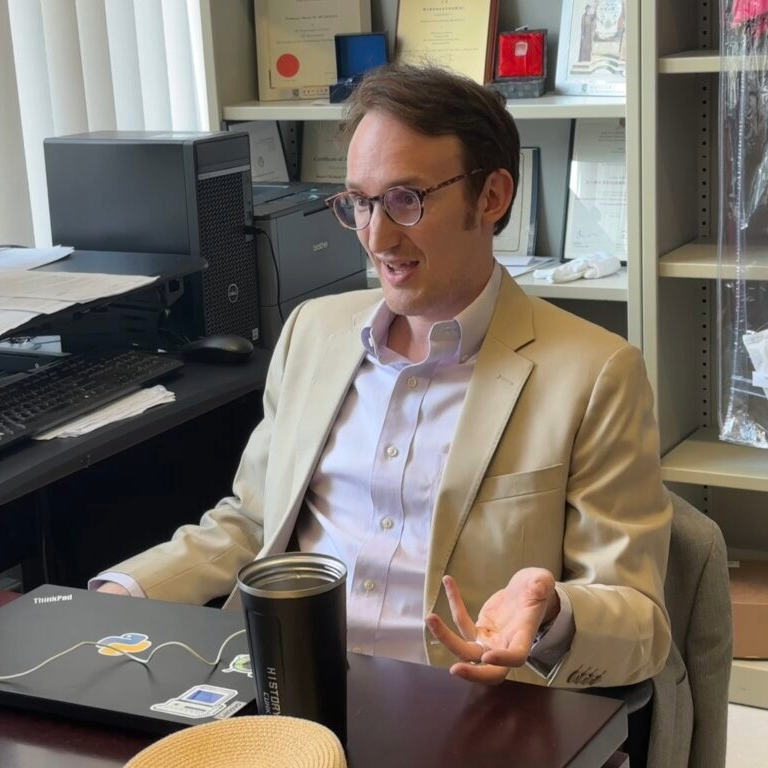
Stories
Dr. Chow Wai Yin Integrates the Research Achievements of Education, Religion and Psychology, Promoting Peace Education and Enhancing the Mental Health of Youth and Communities
Stories
Dr. Chow Wai Yin Integrates the Research Achievements of Education, Religion and Psychology, Promoting Peace Education and Enhancing the Mental Health of Youth and Communities
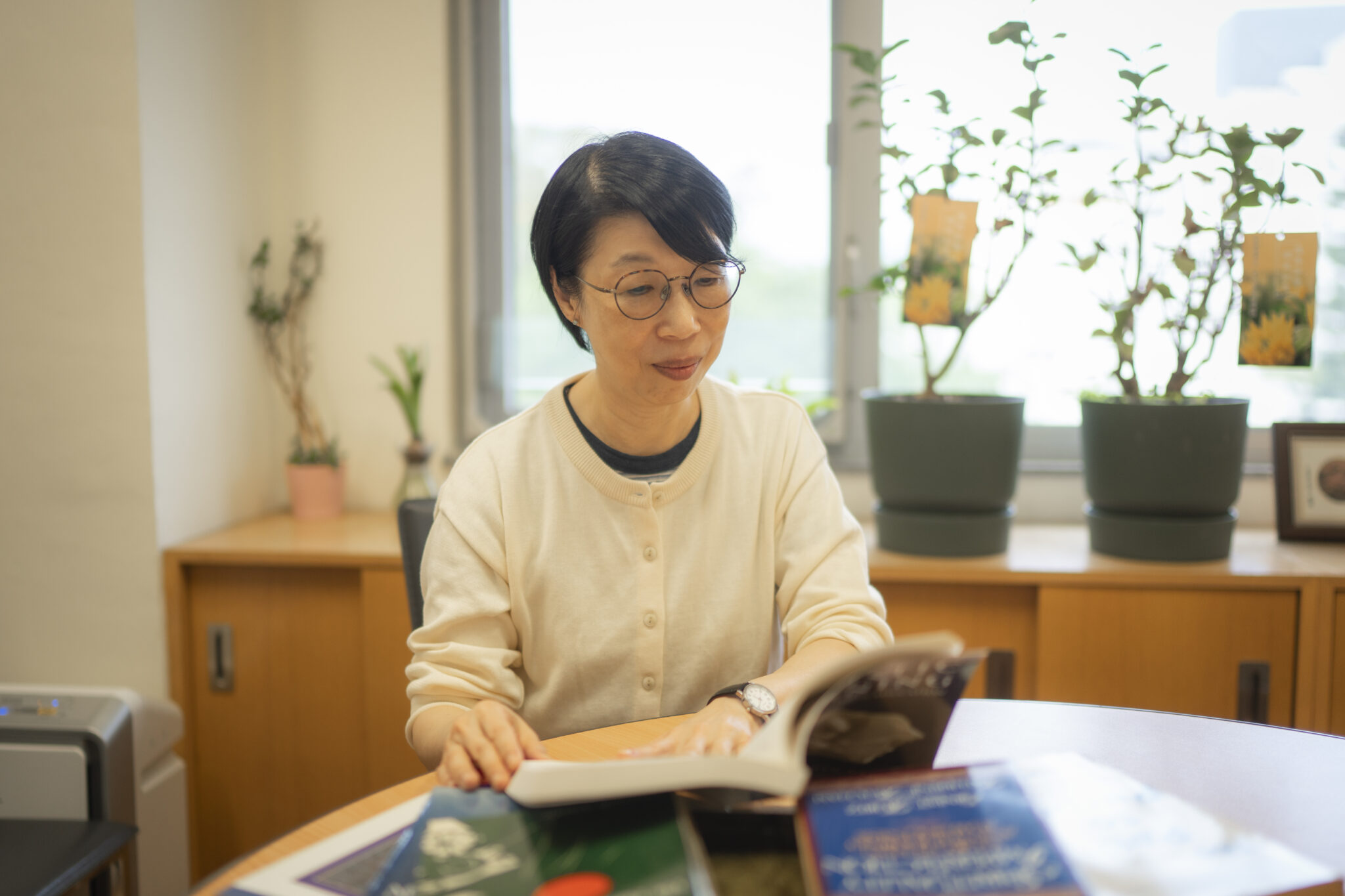
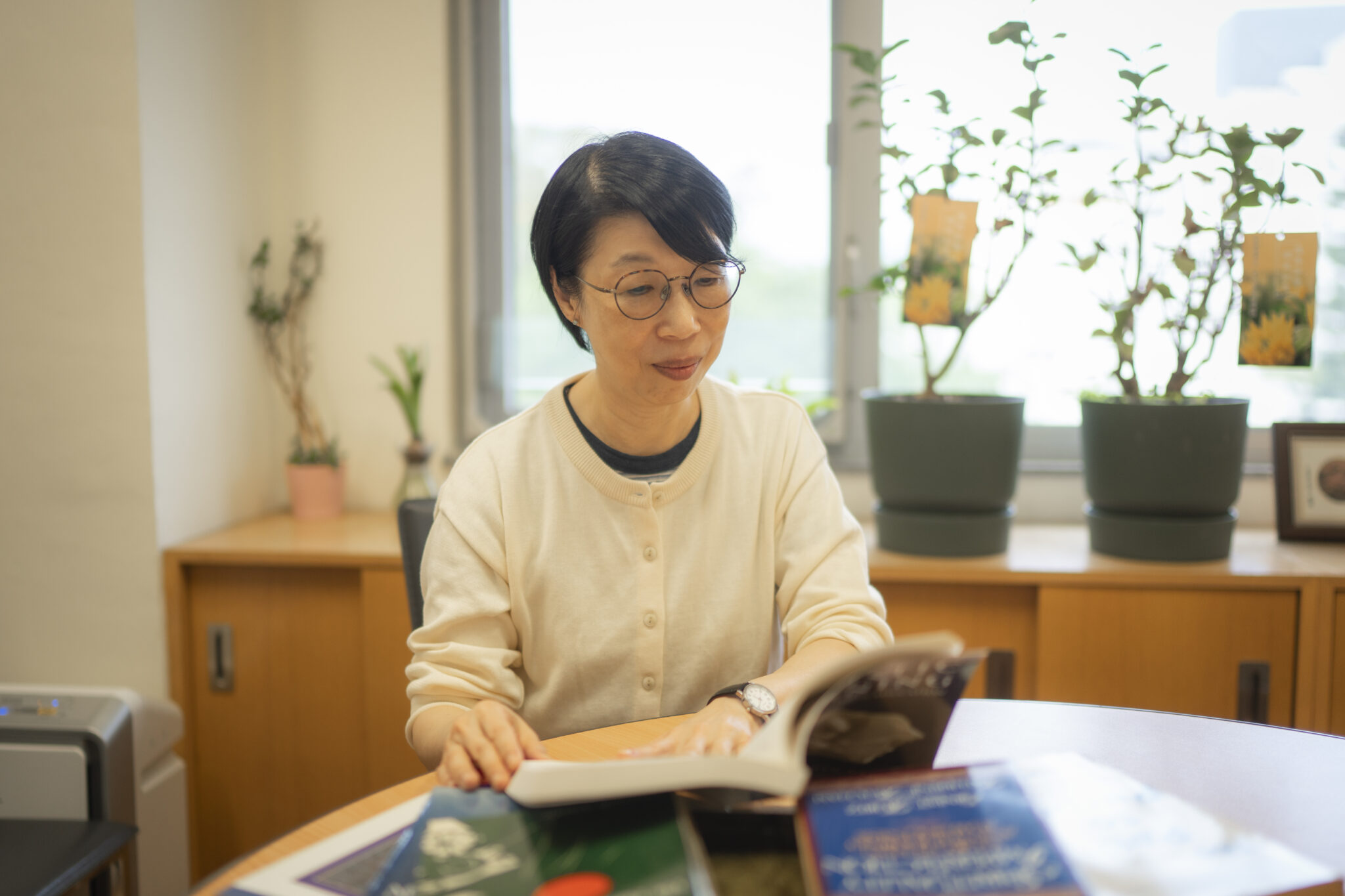

01
04
Latest Update
Explore More
There’s something
more to inspire you.
more to inspire you.
Discover what's current at CUHK ARTS.